Spaces:
Sleeping
Sleeping
import os | |
import pandas as pd | |
import numpy as np | |
import easyocr | |
import streamlit as st | |
from annotated_text import annotated_text | |
from streamlit_option_menu import option_menu | |
from sentiment_analysis import SentimentAnalysis | |
from keyword_extraction import KeywordExtractor | |
from part_of_speech_tagging import POSTagging | |
from emotion_detection import EmotionDetection | |
from named_entity_recognition import NamedEntityRecognition | |
from Object_Detector import ObjectDetector | |
from OCR_Detector import OCRDetector | |
import PIL | |
from PIL import Image | |
from PIL import ImageColor | |
from PIL import ImageDraw | |
from PIL import ImageFont | |
import time | |
# Imports de Object Detection | |
import tensorflow as tf | |
import tensorflow_hub as hub | |
# Load compressed models from tensorflow_hub | |
os.environ['TFHUB_MODEL_LOAD_FORMAT'] = 'COMPRESSED' | |
import matplotlib.pyplot as plt | |
import matplotlib as mpl | |
# For drawing onto the image. | |
import numpy as np | |
from tensorflow.python.ops.numpy_ops import np_config | |
np_config.enable_numpy_behavior() | |
import torch | |
import librosa | |
from models import infere_speech_emotion, infere_text_emotion, infere_voice2text | |
st.set_page_config(layout="wide") | |
hide_streamlit_style = """ | |
<style> | |
#MainMenu {visibility: hidden;} | |
footer {visibility: hidden;} | |
</style> | |
""" | |
st.markdown(hide_streamlit_style, unsafe_allow_html=True) | |
def load_sentiment_model(): | |
return SentimentAnalysis() | |
def load_keyword_model(): | |
return KeywordExtractor() | |
def load_pos_model(): | |
return POSTagging() | |
def load_emotion_model(): | |
return EmotionDetection() | |
def load_ner_model(): | |
return NamedEntityRecognition() | |
def load_objectdetector_model(): | |
return ObjectDetector() | |
def load_ocrdetector_model(): | |
return OCRDetector() | |
sentiment_analyzer = load_sentiment_model() | |
keyword_extractor = load_keyword_model() | |
pos_tagger = load_pos_model() | |
emotion_detector = load_emotion_model() | |
ner = load_ner_model() | |
objectdetector1 = load_objectdetector_model() | |
ocrdetector1 = load_ocrdetector_model() | |
def rectangle(image, result): | |
draw = ImageDraw.Draw(image) | |
for res in result: | |
top_left = tuple(res[0][0]) # top left coordinates as tuple | |
bottom_right = tuple(res[0][2]) # bottom right coordinates as tuple | |
draw.rectangle((top_left, bottom_right), outline="blue", width=2) | |
st.image(image) | |
example_text = "My name is Daniel: The attention to detail, swift resolution, and accuracy demonstrated by ITACA Insurance Company in Spain in handling my claim were truly impressive. This undoubtedly reflects their commitment to being a customer-centric insurance provider." | |
with st.sidebar: | |
image = Image.open('./itaca_logo.png') | |
st.image(image,width=150) #use_column_width=True) | |
page = option_menu(menu_title='Menu', | |
menu_icon="robot", | |
options=["Sentiment Analysis", | |
"Keyword Extraction", | |
"Part of Speech Tagging", | |
"Emotion Detection", | |
"Named Entity Recognition", | |
"Speech & Text Emotion", | |
"Object Detector", | |
"OCR Detector"], | |
icons=["chat-dots", | |
"key", | |
"tag", | |
"emoji-heart-eyes", | |
"building", | |
"book", | |
"camera", | |
"list-task"], | |
default_index=0 | |
) | |
st.title('ITACA Insurance Core AI Module') | |
# Replace '20px' with your desired font size | |
font_size = '20px' | |
if page == "Sentiment Analysis": | |
st.header('Sentiment Analysis') | |
# st.markdown("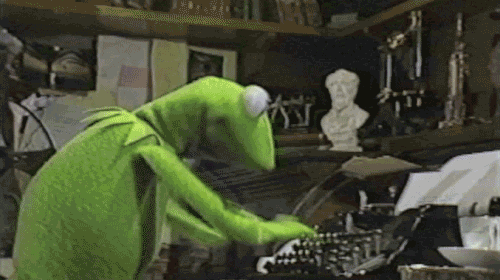") | |
st.write( | |
""" | |
""" | |
) | |
text = st.text_area("Paste text here", value=example_text) | |
if st.button('🔥 Run!'): | |
with st.spinner("Loading..."): | |
preds, html = sentiment_analyzer.run(text) | |
st.success('All done!') | |
st.write("") | |
st.subheader("Sentiment Predictions") | |
st.bar_chart(data=preds, width=0, height=0, use_container_width=True) | |
st.write("") | |
st.subheader("Sentiment Justification") | |
raw_html = html._repr_html_() | |
st.components.v1.html(raw_html, height=500) | |
elif page == "Keyword Extraction": | |
st.header('Keyword Extraction') | |
# st.markdown("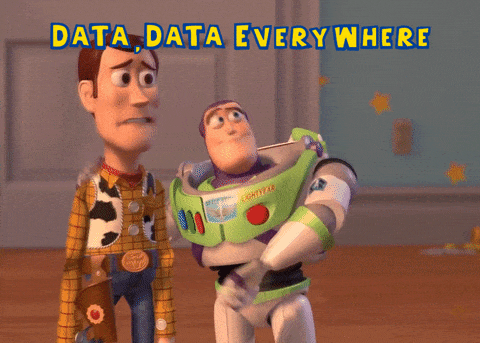") | |
st.write( | |
""" | |
""" | |
) | |
text = st.text_area("Paste text here", value=example_text) | |
max_keywords = st.slider('# of Keywords Max Limit', min_value=1, max_value=10, value=5, step=1) | |
if st.button('🔥 Run!'): | |
with st.spinner("Loading..."): | |
annotation, keywords = keyword_extractor.generate(text, max_keywords) | |
st.success('All done!') | |
if annotation: | |
st.subheader("Keyword Annotation") | |
st.write("") | |
annotated_text(*annotation) | |
st.text("") | |
st.subheader("Extracted Keywords") | |
st.write("") | |
df = pd.DataFrame(keywords, columns=['Extracted Keywords']) | |
csv = df.to_csv(index=False).encode('utf-8') | |
st.download_button('Download Keywords to CSV', csv, file_name='news_intelligence_keywords.csv') | |
data_table = st.table(df) | |
elif page == "Part of Speech Tagging": | |
st.header('Part of Speech Tagging') | |
# st.markdown("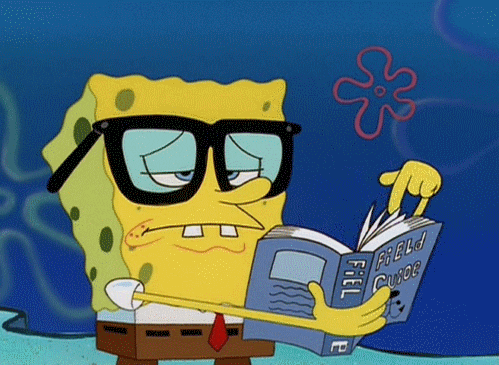") | |
st.write( | |
""" | |
""" | |
) | |
text = st.text_area("Paste text here", value=example_text) | |
if st.button('🔥 Run!'): | |
with st.spinner("Loading..."): | |
preds = pos_tagger.classify(text) | |
st.success('All done!') | |
st.write("") | |
st.subheader("Part of Speech tags") | |
annotated_text(*preds) | |
st.write("") | |
st.components.v1.iframe('https://www.ling.upenn.edu/courses/Fall_2003/ling001/penn_treebank_pos.html', height=1000) | |
elif page == "Emotion Detection": | |
st.header('Emotion Detection') | |
# st.markdown("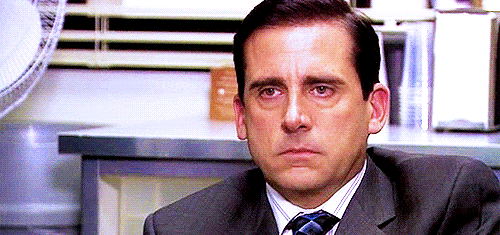") | |
st.write( | |
""" | |
""" | |
) | |
text = st.text_area("Paste text here", value=example_text) | |
if st.button('🔥 Run!'): | |
with st.spinner("Loading..."): | |
preds, html = emotion_detector.run(text) | |
st.success('All done!') | |
st.write("") | |
st.subheader("Emotion Predictions") | |
st.bar_chart(data=preds, width=0, height=0, use_container_width=True) | |
raw_html = html._repr_html_() | |
st.write("") | |
st.subheader("Emotion Justification") | |
st.components.v1.html(raw_html, height=500) | |
elif page == "Named Entity Recognition": | |
st.header('Named Entity Recognition') | |
# st.markdown("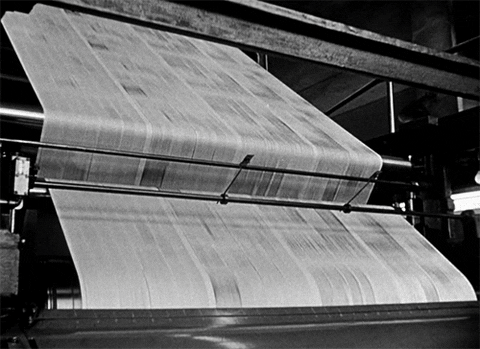") | |
st.write( | |
""" | |
""" | |
) | |
text = st.text_area("Paste text here", value=example_text) | |
if st.button('🔥 Run!'): | |
with st.spinner("Loading..."): | |
preds, ner_annotation = ner.classify(text) | |
st.success('All done!') | |
st.write("") | |
st.subheader("NER Predictions") | |
annotated_text(*ner_annotation) | |
st.write("") | |
st.subheader("NER Prediction Metadata") | |
st.write(preds) | |
elif page == "Object Detector": | |
st.header('Object Detector') | |
st.write( | |
""" | |
""" | |
) | |
img_file_buffer = st.file_uploader("Load an image", type=["png", "jpg", "jpeg"]) | |
if img_file_buffer is not None: | |
image = np.array(Image.open(img_file_buffer)) | |
if st.button('🔥 Run!'): | |
with st.spinner("Loading..."): | |
img, primero = objectdetector1.run_detector(image) | |
st.success('The first image detected is: ' + primero) | |
st.image(img, caption="Imagen", use_column_width=True) | |
elif page == "OCR Detector": | |
st.header('OCR Detector') | |
st.write( | |
""" | |
""" | |
) | |
file = st.file_uploader("Load an image", type=["png", "jpg", "jpeg"]) | |
#read the csv file and display the dataframe | |
if file is not None: | |
image = Image.open(file) # read image with PIL library | |
if st.button('🔥 Run!'): | |
with st.spinner("Loading..."): | |
result = ocrdetector1.reader.readtext(np.array(image)) # turn image to numpy array | |
# collect the results in dictionary: | |
textdic_easyocr = {} | |
for idx in range(len(result)): | |
pred_coor = result[idx][0] | |
pred_text = result[idx][1] | |
pred_confidence = result[idx][2] | |
textdic_easyocr[pred_text] = {} | |
textdic_easyocr[pred_text]['pred_confidence'] = pred_confidence | |
# get boxes on the image | |
rectangle(image, result) | |
# create a dataframe which shows the predicted text and prediction confidence | |
df = pd.DataFrame.from_dict(textdic_easyocr).T | |
st.table(df) | |
elif page == "Speech & Text Emotion": | |
st.header('Speech & Text Emotion') | |
st.write( | |
""" | |
""" | |
) | |
uploaded_file = st.file_uploader("Choose an audio file", type=["mp3", "wav", "ogg"]) | |
if uploaded_file is not None: | |
st.audio(uploaded_file, format='audio/' + uploaded_file.type.split('/')[1]) | |
st.write("Audio file uploaded and playing.") | |
else: | |
st.write("Please upload an audio file.") | |
if st.button("Analysis"): | |
with st.spinner("Loading..."): | |
st.header('Results of the Audio & Text analysis:') | |
samples, sample_rate = librosa.load(uploaded_file, sr=16000) | |
p_voice2text = infere_voice2text (samples) | |
p_speechemotion = infere_speech_emotion(samples) | |
p_textemotion = infere_text_emotion(p_voice2text) | |
st.subheader("Text from the Audio:") | |
st.write(p_voice2text) | |
st.write("---") | |
st.subheader("Speech emotion:") | |
st.write(p_speechemotion) | |
st.write("---") | |
st.subheader("Text emotion:") | |
st.write(p_textemotion) | |
st.write("---") | |